Introduction
The journey from AI conception to production is fraught with challenges, a reality reflected in the very low proportion of AI projects make it from pilot to production. Central to these challenges are two pivotal aspects: data quality and model operations (MLOps). Poor data quality can significantly hinder AI model accuracy and performance, while inefficient model operations impede the transition from development to deployment. Addressing these key areas is crucial for accelerating the success rate of AI projects. This article aims to unpack these complexities, shedding light on how Kili Technology and CraftAI work together to help companies advance and succeed in their AI projects.
Importance of Data Quality
High-quality data is essential for training accurate and effective machine learning models. Poor data quality can lead to incorrect or biased results, significantly diminishing the model's performance. The phrase “Garbage In, Garbage Out” remains true in AI regarding data, the quality of the input data directly impacts the reliability and validity of a model’s results.
Studies indicate that a mere 10% decline in labeling accuracy can lead to a significant 2 to 5% decrease in model performance. Such a decline affects the functionality of AI systems and escalates costs due to the need for larger, more complex datasets.
However, achieving high-quality data is challenging, and presents a bottleneck for developing well-performing models. Even popular public datasets have significant error rates, underlining the complexity of accurate data labeling.
Challenges in Achieving High-Quality Data
Data labeling, a critical step in training machine learning models, presents several challenges due to its complex and multifaceted nature.
Accuracy and Consistency

Achieving high accuracy in data labeling is paramount, as even minor errors can significantly impact the performance of the resulting model. Maintaining consistency across large datasets adds complexity, especially when multiple annotators are involved. Ensuring that every labeler understands and applies the criteria similarly is challenging.
Complexity of Data and Tasks
Depending on the nature of the project, data labeling can involve intricate tasks such as identifying nuanced emotional cues in text, delineating objects in images with high precision, or dealing with highly specialized domain-specific content. Some labeling tasks are inherently subjective, leading to variability in how different annotators interpret the same data. This is particularly true for tasks like sentiment analysis or certain types of classification, where personal biases and perceptions can influence the labeling process. This complexity demands expertise that can be hard to find and expensive to employ.
Volume and Scalability

Machine learning models, particularly those based on deep learning, require large amounts of labeled data. Handling such volumes can be resource-intensive and time-consuming. Scaling the labeling process while maintaining quality and efficiency is a non-trivial task that often requires a combination of automated tools and human oversight.
Evolving Data and Standards
Data labeling is not a one-time activity. As models evolve and new data comes in, labels might need to be updated or expanded. Keeping up with these changes without disrupting the ongoing processes requires a flexible and adaptive approach.
How Kili Technology solves data quality challenges
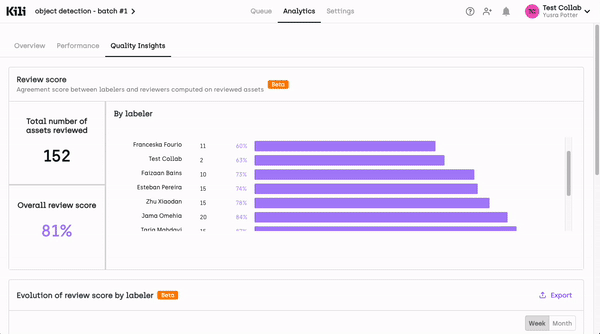
Through its advanced data labeling platform, Kili Technology addresses data quality challenges in AI and machine learning.
First, Kili Technology streamlines the data labeling process, by enhancing a labeler’s experience through an efficient and user-friendly platform. It offers various productivity-enhancing features, such as keyboard shortcuts and ML automated labeling functions to help scale data labeling operations. These functions include model-in-the-loop, GPT-automated text labeling, efficient object detection based on the SAM model, and more.
Kili Technology also emphasizes the importance of data quality right from the start. The platform includes robust quality assurance features, ensuring the data is accurately labeled and meets the required standards for AI model training. It allows for feedback and collaboration, efficient random and targeted reviews, integrated quality metrics, programmatic QA for fast error detection, and consensus and honeypot methods to ensure data labeling with the highest accuracy and speed.
Revolutionize Your AI Journey: The Power of Mastering MLOps
In the ever-evolving realm of Artificial Intelligence (AI), the transition from concept to production presents an intricate challenge. The success of AI projects relies heavily on the ability of companies to deploy reliable applications swiftly and at a minimal cost. We will unveil how mastering MLOps, a groundbreaking approach inspired by agile methodologies and devOps tools, can dramatically enhance AI project management and lead to success in a sector where a staggering 80% of projects do not reach fruition.
Embracing the Agile AI Shift with MLOps
In the coming LLMs era, traditional linear workflows are becoming obsolete. The complexity of AI models and the sheer volume of data necessitate a shift towards more agile and iterative development methods. This approach, segmenting development into short, regular cycles, is critical for maximizing results and reducing risks. Embracing this agile AI strategy is essential for anyone looking to make significant strides in the AI field. So we need a technology that can take companies from prototype to production in a very short space of time and with limited resources: that's where MLOps comes in.
Efficient AI Deployment with MLOps Integration

Adopting MLOps at the beginning of your AI project equips your organization with an advanced toolkit for efficient project management. This strategy not only cuts costs and boosts project outcomes but also improves model quality and simplifies processes, greatly diminishing the likelihood of failure. It fosters a cohesive environment among all teams involved, setting a solid foundation for successful AI deployment.
Constructing Your MLOps Workflow: A Beginner's Guide
AI projects often start in the realm of a Data Scientist, yet their successful deployment requires the concerted efforts of ML Engineers, DevOps, and developers. MLOps offers the essential tools and practices for a unified and cost-effective project management approach. This methodology enables early prototyping, API service testing, and the gathering of valuable feedback from end users, thereby establishing a cycle of continuous improvement and involvement.
Reduce 80% the time of DevOps and 25% the time of Data Science

One of the key benefits of MLOps is its ability to create economies of scale and immediate financial gains. By optimizing both human and machine resources, MLOps achieves significant cost reductions. Furthermore, it could also lead to Cloud Resource Optimization, potentially saving up to 20% in infrastructure costs using resources in a more rational and optimised way. Furthermore, reducing the average deployment time from months to mere minutes not only lowers production costs but also boosts revenues, improving the financial trajectory of AI projects.
Simplifying Deployment with a User-Friendly Interface

A significant advantage of MLOps is the simplicity it brings to project management. An all-encompassing platform or coherent stack eases the onboarding process, streamlines iteration management, and enhances internal collaboration. The high reproducibility of pipelines prevents task duplication, elevating project quality and maintenance ease. Early and transparent results also promote better adoption among business teams, increasing end-user trust and satisfaction.
How Craft AI solves MLOps challenges
Craft AI's MLOps platform addresses the challenges of deploying and managing machine learning models by offering a seamless, time-saving product designed for collaboration. Craft AI's solution simplifies the transition from proof of concept to production, automating all the steps in the ML workflow to reduce development time and enhance model reliability. It allows users to easily create environments on various cloud platforms without needing advanced DevOps skills, supporting both CPU and GPU computing. The platform is versatile, enabling the deployment of Python scripts with a wide array of models, LLM, and libraries like PyTorch and SKlearn. It focuses on creating scalable, fully monitored, and production-ready applications with complete logs and analytics.
The platform also adopts a responsible AI approach, addressing Large Language Model (LLM) hallucination issues and optimizing infrastructure costs. It enables private usage on dedicated servers, balancing efficiency and privacy. Finally, the platform supports regulation compliance like GDPR or AI act, offering a comprehensive and user-friendly solution for regulation challenges.
In a nutshell, Craft AI is a platform dedicated to the rapid, high-performance industrialisation of trusted AI.
The magic of high-quality data and a powerful MLOps platform
As we've explored, the challenges of achieving high-quality data are numerous, but with Kili Technology's advanced data labeling platform, these hurdles are effectively addressed. Similarly, integrating a powerful MLOps platform, like that offered by Craft AI, transforms the landscape of AI project management, making the journey from concept to production seamless and efficient.
The partnership between Kili Technology and Craft AI marks a significant step in practical AI development. This collaboration addresses two critical areas in AI projects: high-quality data and efficient project management.
Data Quality and MLOps Synergy: Kili Technology's role in enhancing data quality complements Craft AI's focus on efficient MLOps. This synergy simplifies the process from data preparation to model deployment.
Addressing Key AI Challenges: By combining Kili's data labeling accuracy with Craft AI's agile deployment strategies, the partnership effectively tackles common obstacles in AI project development, such as data errors, deployment delays, and resource inefficiencies.
Cost and Time Efficiency: The collaboration aims to reduce the time and costs associated with AI projects. Streamlined data labeling and project management processes are central to achieving this goal.
Future-Readiness: As AI evolves, the importance of data integrity and agile methodologies becomes more pronounced. This partnership equips companies with the tools to stay relevant in a rapidly advancing field.
Real-World Application: The joint effort is designed to have a tangible impact, translating AI potentials into real-world applications and outcomes.
In conclusion, using Kili Technology and Craft AI is a practical response to the demands of modern AI development. The platforms offer a more streamlined, efficient pathway for turning AI concepts into viable solutions. For organizations seeking to improve their AI project outcomes, exploring this collaboration could be a step toward more efficient and effective AI development.
Take the next steps for successful AI deployment:
Don't let the complexities of data quality and MLOps hinder your AI ambitions. Instead, harness the power of this formidable partnership to streamline your AI development process and achieve remarkable results.
Discover firsthand how the advanced data labeling capabilities of Kili Technology, combined with the agile MLOps solutions of CraftAI, can revolutionize your AI projects. A demo will provide an in-depth look at how these platforms work in tandem to overcome common AI development challenges. Reach out to our teams today!