This article was originally published on The Conversation website.
Today, this dream is almost becoming reality with the project Schopper supported by the National Research Agency. Five French partners revolve around it: three laboratories (CERP-HNHP, CEROS and LIX) and two companies (Craft.AI and Immersion Tools) who together create innovative technological solutions applied to archaeological research.
In particular, this project allowed us to arrive at a technology generating the landscapes of the Tautavel Valley frequented by prehistoric men during contrasting climatic periods (glacial and interglacial), between 600,000 years and 90,000 years before the present.
The simulation is powered by climate parameters (temperature, humidity) obtained by models of Machine learning (machine learning) applied to past periods. It makes it possible to position plant species according to their ecological abilities and animals that move and feed according to available resources and their ethology.
Combined with the development of the entire valley in immersive 3D, the result now offers archaeological researchers the possibility of moving at a 1:1 scale in the valley in order to appreciate the relief of the terrain and the distances, the density of the plant cover, the areas of crossing natural barriers, the areas of crossing natural barriers, of grouping and of passage of animals. These are all important points of reference for understanding the mobility of hunter-gatherers. It is also possible to observe the arrangements of flora whose pollens have been found fossilized in the cave, or to follow the evolution of the landscape.
54 years of excavations
At the origin of this virtual reconstruction, we find “Schopper”, a simulator that makes it possible to test hypotheses about the environment and the behaviors of prehistoric men in a reconstructed immersive environment. The principle is first to learn from archaeological data, to then formulate hypotheses about behavior or the environment, and finally to observe the mechanisms and impacts of these hypotheses in the reconstituted environment.
This simulator is the result of two interacting platforms.
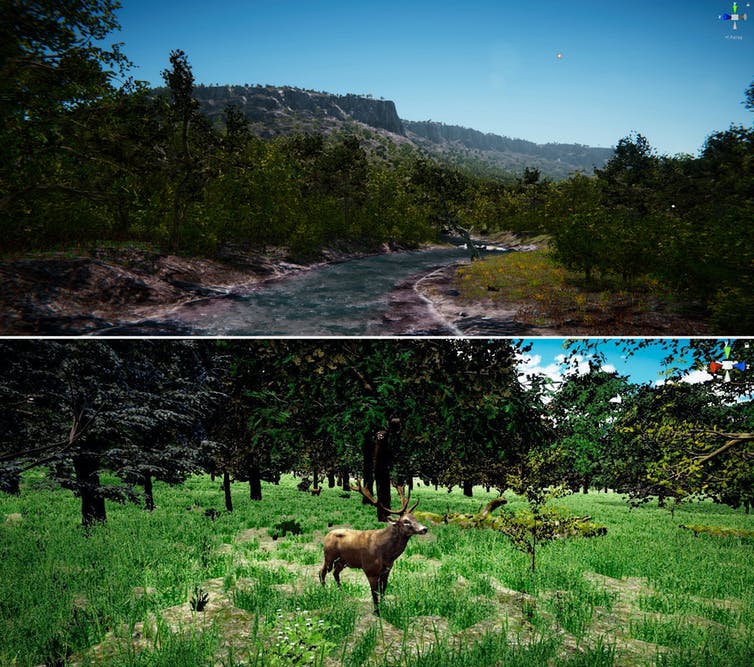
The first is based on the database of the prehistory research laboratory located in Tautavel, in charge of excavating the pilot site of the project, the Caune de l'Arago. This Lower Paleolithic site of global interest has delivered, among other things, the oldest human fossils on French territory.
Thanks to the work of the prehistorian Henry de Lumley, the CERP has built up a database that memorizes 54 years of excavations using a structured methodology. It contains nearly 500,000 objects (animal bones, lithic industries, etc.), corresponding to about fifty times when the cave was occupied, as well as samples (sediments, pollens, etc.).
To exploit this database, Craft.AI, a start-up specializing in artificial intelligence (AI), has developed an engine for Schopper that makes it possible to test scientific hypotheses. It is thus possible to question, for example, the duration of the periods of occupation of the cave, the function it had for the men of the past, but also the climatic conditions.
The second platform is created by the Immersion Tools team, which specializes in the integration of innovative visual presentation tools. It offers archaeologists the possibility of interacting in virtual reality, in immersion, with the database in the cave modeled in 3D as shown in the animation below.
Each object is materialized by a parallelepiped of color corresponding to its nature. Their spatial position at the time of their discovery during the dig, their orientation and their inclination are respected. Researchers have access to a range of tools that allow them to measure distances between objects, to display 3D scans or the grid, or to move around by following body movements or by “teleportation”.
Two approaches to training AI
To work, an AI tool needs to learn. When it comes to supervised learning, as is the case with Schopper, it is necessary to give him “labeled” data, for example combining a set of remains of flora and fauna with a certain climate.
Two major difficulties arise here in archaeology. First of all, the data volume is low. The data comes from several academic disciplines and is therefore quite heterogeneous. They remain even more difficult to interpret: as no one was there 400,000 years ago to find out whether it was hot or cold, it seems difficult to know under what climatic conditions a plant whose pollen fossil we can find developed.
So we had to adapt AI training modes to these specific constraints of archaeology. The first training mode proposed in Schopper is thus based on “actualism”: it is a question of admitting that what is happening now is similar to what happened a long time ago (in some cases). This allows us to have access to a greater volume of data by enriching prehistoric data with current data.
For example, it is assumed that the reindeer hunted by Tautavel's man 450,000 years ago has the same ecology as the current reindeer. This is tantamount to hypothesizing that he lived in a relatively cold climate in Arctic or subarctic regions. The holm oak, whose pollen grains are taken from certain levels of the Caune de l'Arago, should remain typical of the current Mediterranean region, thermophilic and resistant to drought.
For wildlife, we refer in particular to an important WWF database listing vertebrate species from all ecoregions of the world. These represent data points that nourish learning by associating animals with the characteristics of their environment. It may be the Biome terrestrial, an annual average temperature value, or even a total of precipitations in millimeters over the year.

The second mode used has as its starting point “expert opinions”. An archaeologist, depending on his specialty, will for example deduce from a set of data that men at a certain date had only lived briefly in the cave.
The AI then questions the same elements to identify those who, in its opinion, prompted the researcher to give this opinion. Moreover, it may happen that the algorithm infers that the variables decisive in the final decision differ from those stated by the expert in his articles.
Exploitation of models
Once the data is prepared in this way, a series of round trips begins in order to identify the optimal parameters. It is interspersed with validation steps to determine the quality of the model's learning as well as its generalization power. In this sense, machine learning follows the Principle of Ockham's razor where simpler modeling is preferred to an overly complex explanation.
The models are finally being applied to understand, in the Caune de l'Arago region and at different times, the biome, the type of climate, the temperature, the quantity of precipitation or the duration of occupancy and the function of the site.
Of Explanation algorithms such as SHAP are also used to understand how one model leads to one decision and not another. In particular, this allows archaeologists who are not experts in machine learning to understand the decision-making processes implemented in the models they use.
Now it remains to deepen the treatment by the model of what affects the behaviors of our ancestors. Unfortunately, this comes up against the difficulties of establishing solid learning frameworks with little data on such ancient periods. However, the project consortium is working on new technical approaches to improve the performance of AI and add immersion through sound. This will be the continuation of Schopper's developments.
This paper was written with Philippe Carrez, founder of Immersion-Tools, and Matthieu Boussard, Research and Development engineer at Craft AI, two partners in the Schopper project.