“Artificial intelligence is when the machine tries to do what humans do, but not as well.”
This definition, which might seem counterintuitive or even grossly iconoclastic, is in fact one of the most precise there is. It makes it easy to separate what is and is not AI in order to replicate human cognitive processes. For example, a super calculator is not AI, a computer will always be better than a human at doing a mathematical calculation. On the other hand, software that is capable of systematically recognizing a person in photos contains AI because we are typically in the situation where a human is better at this exercise. If an AI is systematically less efficient in its task than a human could be, what is its interest? The ladder crossing! What a human does perfectly, an AI will do almost as well, but more importantly, will do it millions of times more in the same time. A great calculator whose calculations make it possible to recognize photos, here is one of the countless AI use cases that are part of our daily lives.
The deployment of AI solutions is made possible thanks to the multiplication of the data we produce and the explosion of the computing power of our equipment. This Datafication of society as well as recent algorithmic maturity allow a decisive shift for companies: moving from a “rear-view mirror” management that prevailed until now (learning from the past) to a predictive vision.
To better understand how our data makes predictions possible, we must first discern the four levels of data analysis: observe, understand, predict, and prescribe.
- Observer: the famous rear-view mirror that makes it possible to identify what happened through a detailed analysis of historical data.
- Understand: the higher level of analysis that allows you to draw concrete lessons from data in order to understand what happened.
- Predicting: the first level of analysis no longer focused on the past but on the future: being able to predict in a probabilistic way what will happen.
- Prescribe: the latest level of data analysis, it allows businesses to make the best decision by taking advantage of what they have been able to learn.

Predictive and prescriptive levels are made possible by the advent of tools for Machine Learning (machine learning). The concept of Machine Learning is based on three phases: learning (the machine recognizes patterns and correlations in historical data), the inference phase (the machine applies what it has learned), and the supervision phase (Managing the life cycle, model performance over time and potential retraining).
These models of Machine Learning allow businesses today to move into the predictive era and deploy operational AI solutions of three types: forecasting & optimization, hyper-personalization and automation.
Forecasting & optimization
Precisely anticipate the needs, risks and failures of an industrial process in order to implement appropriate actions in the right place at the right time.
Example: Predictive maintenance (Industry)
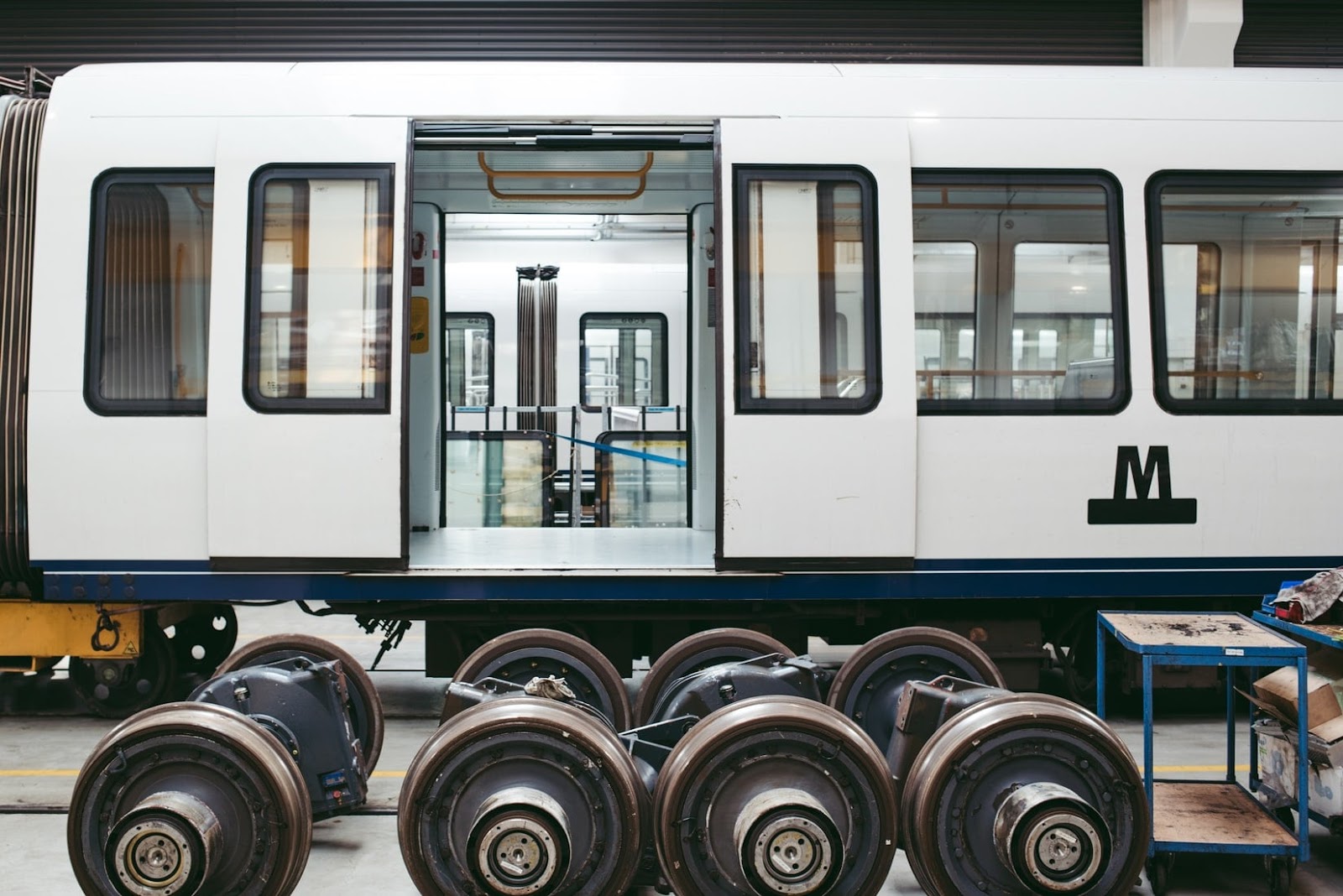
Detect structural defects, premature wear and loss of machine performance in order to prioritize maintenance operations and maximize production.
Hyper-personalization
Anticipate the specific needs of a user based on their data and make tailor-made proposals to help them on a daily basis.
Example: Personalized learning (Education)
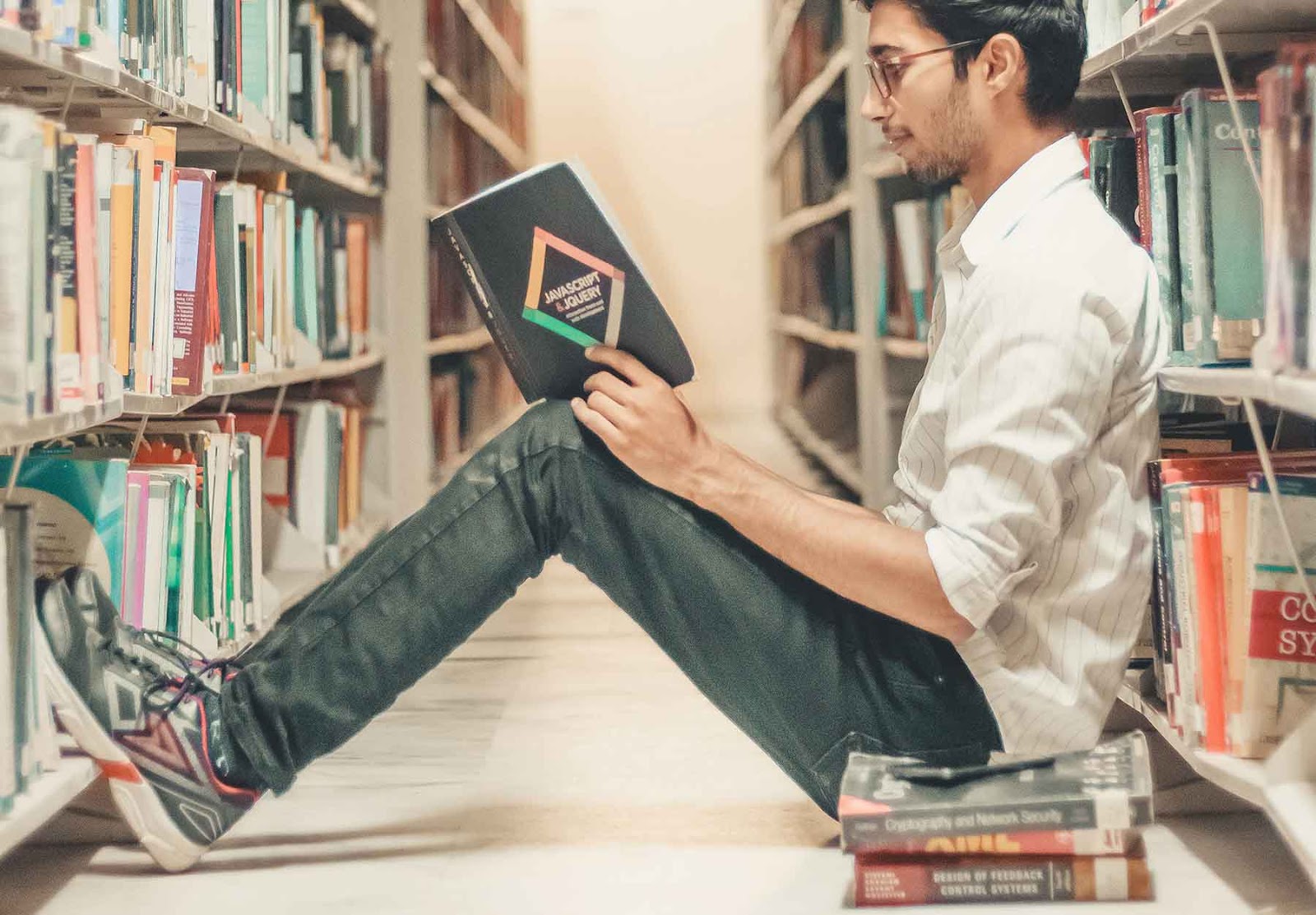
The purpose of this application is to support students in the manner of a virtual tutor and to recommend content to them based on their background, their cognitive profile and their pedagogical shortcomings.
Automation
Relieve employees of thankless tasks that require numerous iterations without the human having any added value in the process.
Example: Energy management of a building stock (Energy)

The objective is to develop an energy manager that automates the supervision of a building stock, tracks any consumption drift and forecasts it to improve overall energy efficiency.
At Craft AI, we are thus deploying more than a dozen use cases in key sectors of our society such as energy, education or even health.
This is possible because we are approaching maturity on the algorithmic part and are in a position to collect enough data. However, a third ingredient is missing to really talk about revolution: use.
There is a deficit in the use and adoption of AI in businesses, as noted by Gartner in a study : 85% of AI projects do not go into production and do not generate any ROI. There are two main reasons that can explain this lack of adoption:
- The difficulty of industrializing AI projects (The famous industrialization wall)
- Society's distrust of AI, the fear of seeing machines make decisions for us.
If they are well addressed, one by the MLops, the other by one Trusted AI, you can very easily develop multiple application cases and fully enter the predictive era.